Transformation-Grounded Image Generation Network for Novel 3D View Synthesis
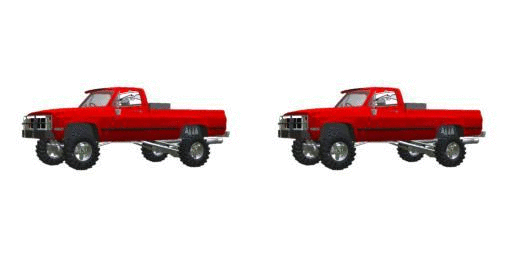
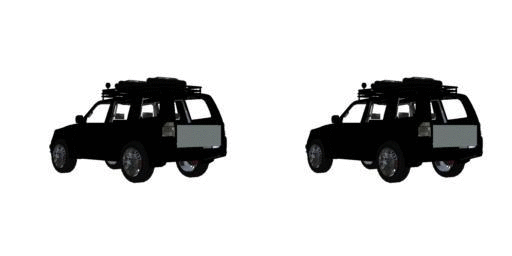
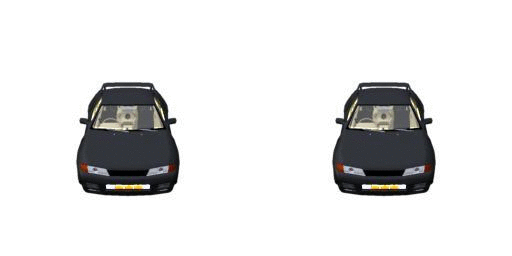
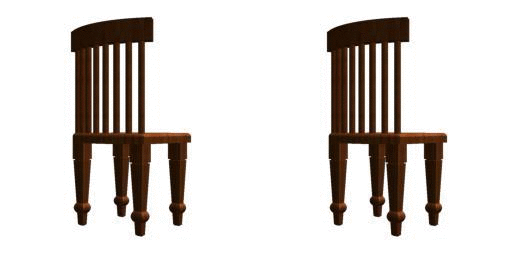
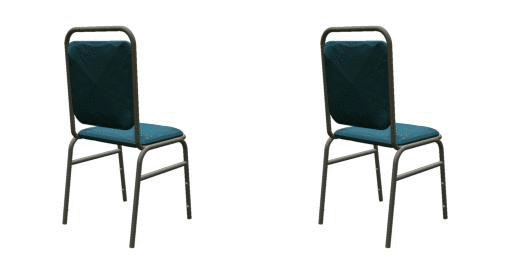
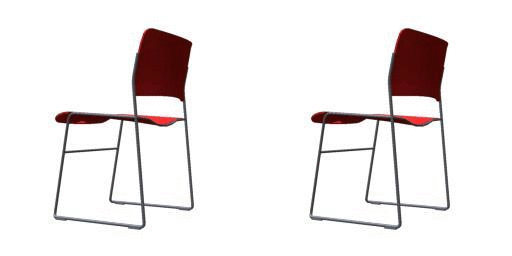
[1st-Input View Images, 2nd-Synthesized Images]
Abstract
We present a transformation-grounded image generation network for novel 3D view synthesis from a single image. Instead of taking a 'blank slate' approach, we first explicitly infer the parts of the geometry visible both in the input and novel views and then re-cast the remaining synthesis problem as image completion. Specifically, we both predict a flow to move the pixels from the input to the novel view along with a novel visibility map that helps deal with occulsion/disocculsion. Next, conditioned on those intermediate results, we hallucinate (infer) parts of the object invisible in the input image. In addition to the new network structure, training with a combination of adversarial and perceptual loss results in a reduction in common artifacts of novel view synthesis such as distortions and holes, while successfully generating high frequency details and preserving visual aspects of the input image. We evaluate our approach on a wide range of synthetic and real examples. Both qualitative and quantitative results show our method achieves significantly better results compared to existing methods.
Transformation-Grounded View Synthesis Network(TVSN)
Given an input image and a target transformation, our disocclusion-aware appearance flow network (DOAFN) transforms the input view by relocating pixels that are visible both in the input and target view. The image completion network, then, performs hallucination and refinement on this intermediate result. For training, the final output is also fed into two different loss networks in order to measure similarity against ground truth target view

Qualitative Results
[1st-Input, 2nd-Ground Truth, 3rd-Appearance Flow Network, 4th-Predicted visibility maps, 5th-Disocclusion-aware appearance flow network, 6th-TVSN]
Car
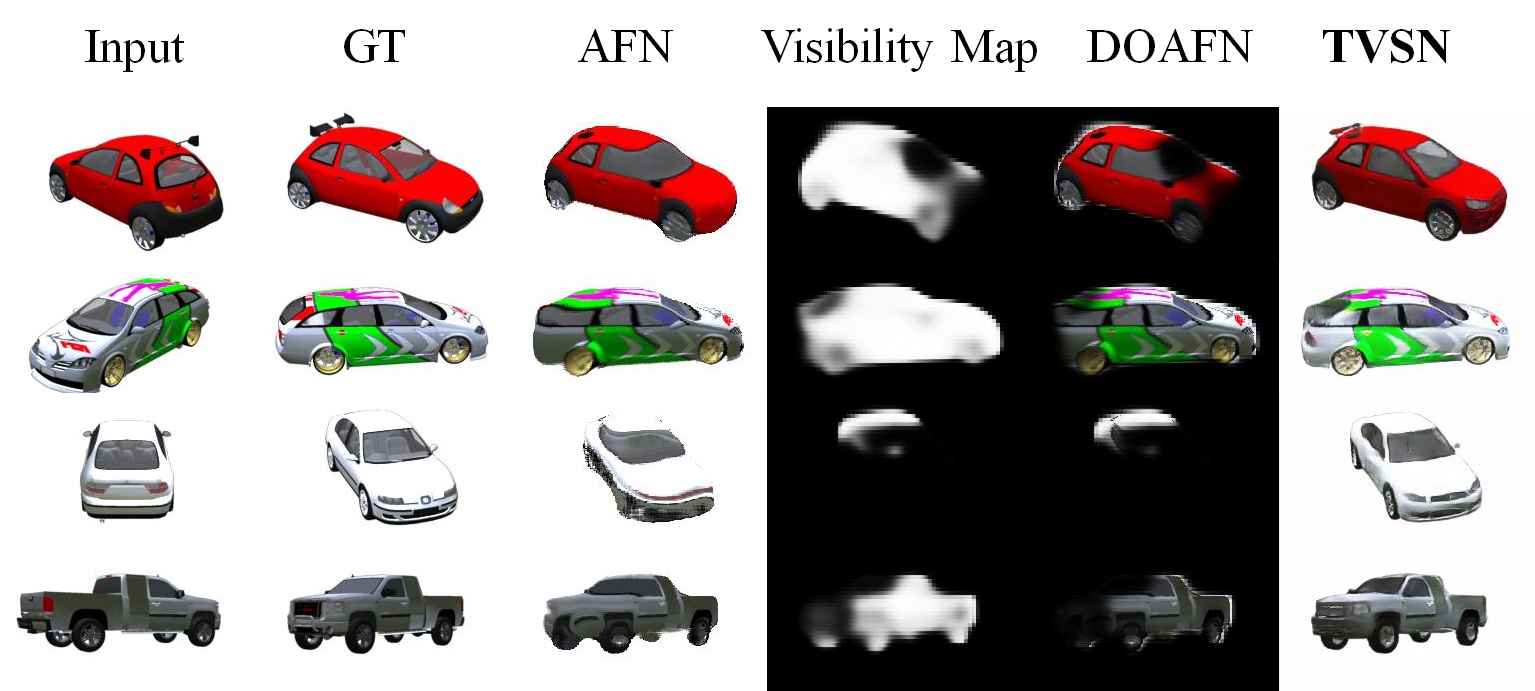
Chair
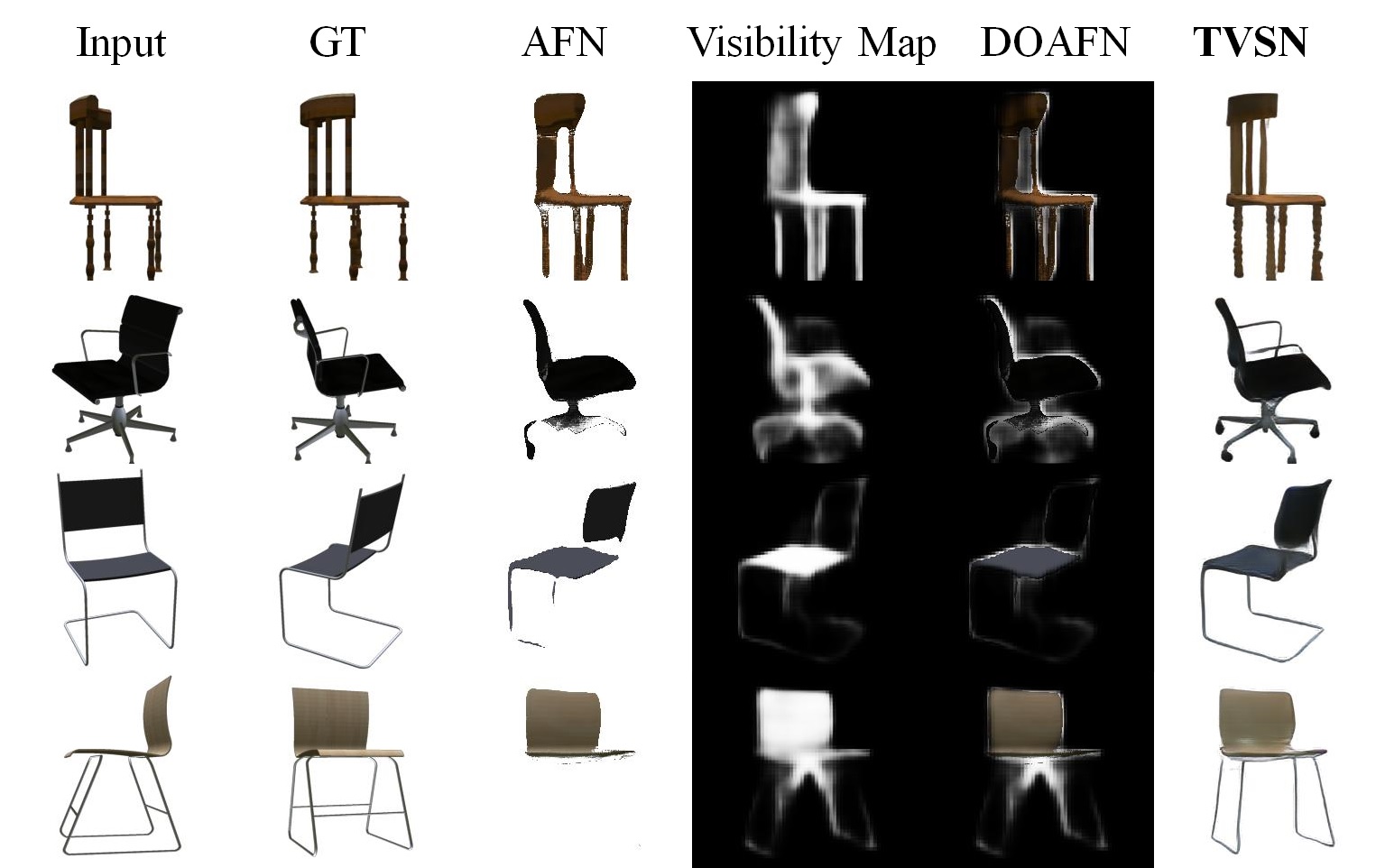
Motorcycle
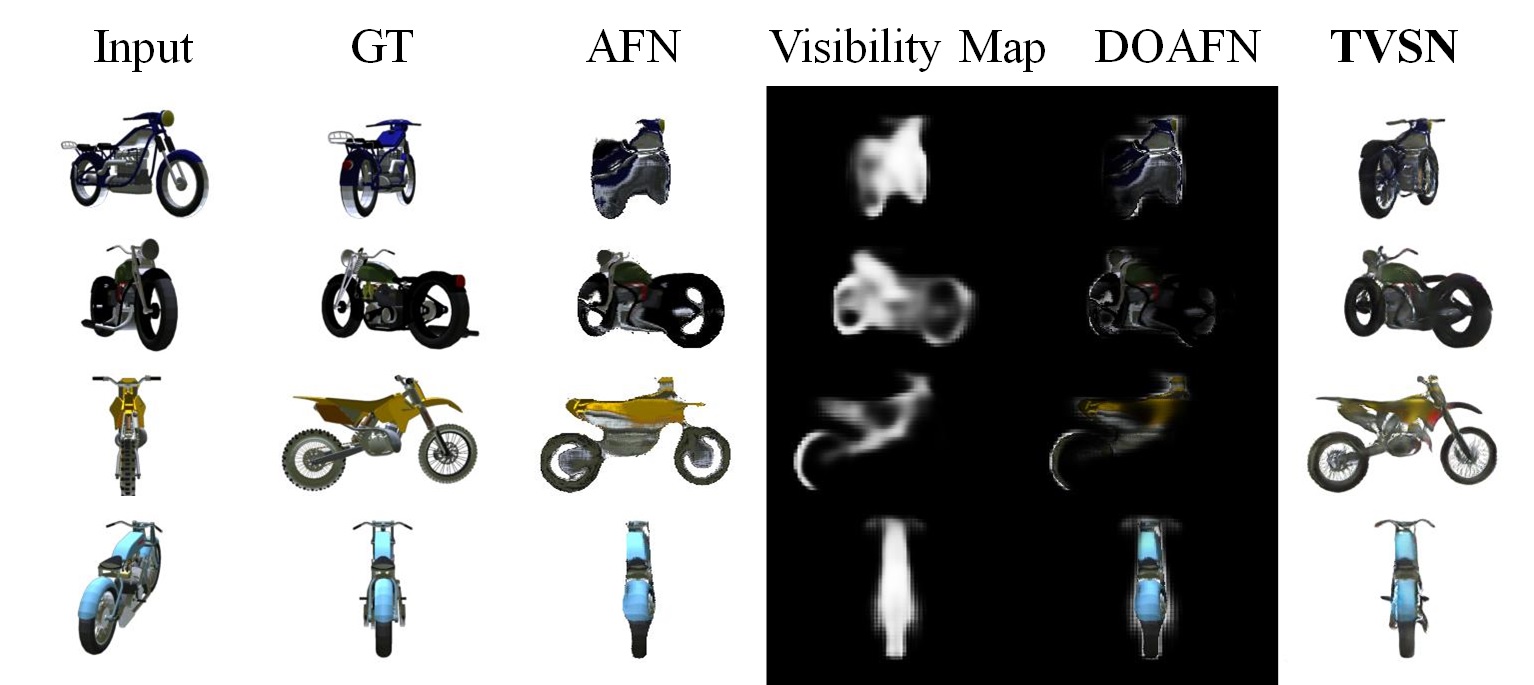
Flowerpot
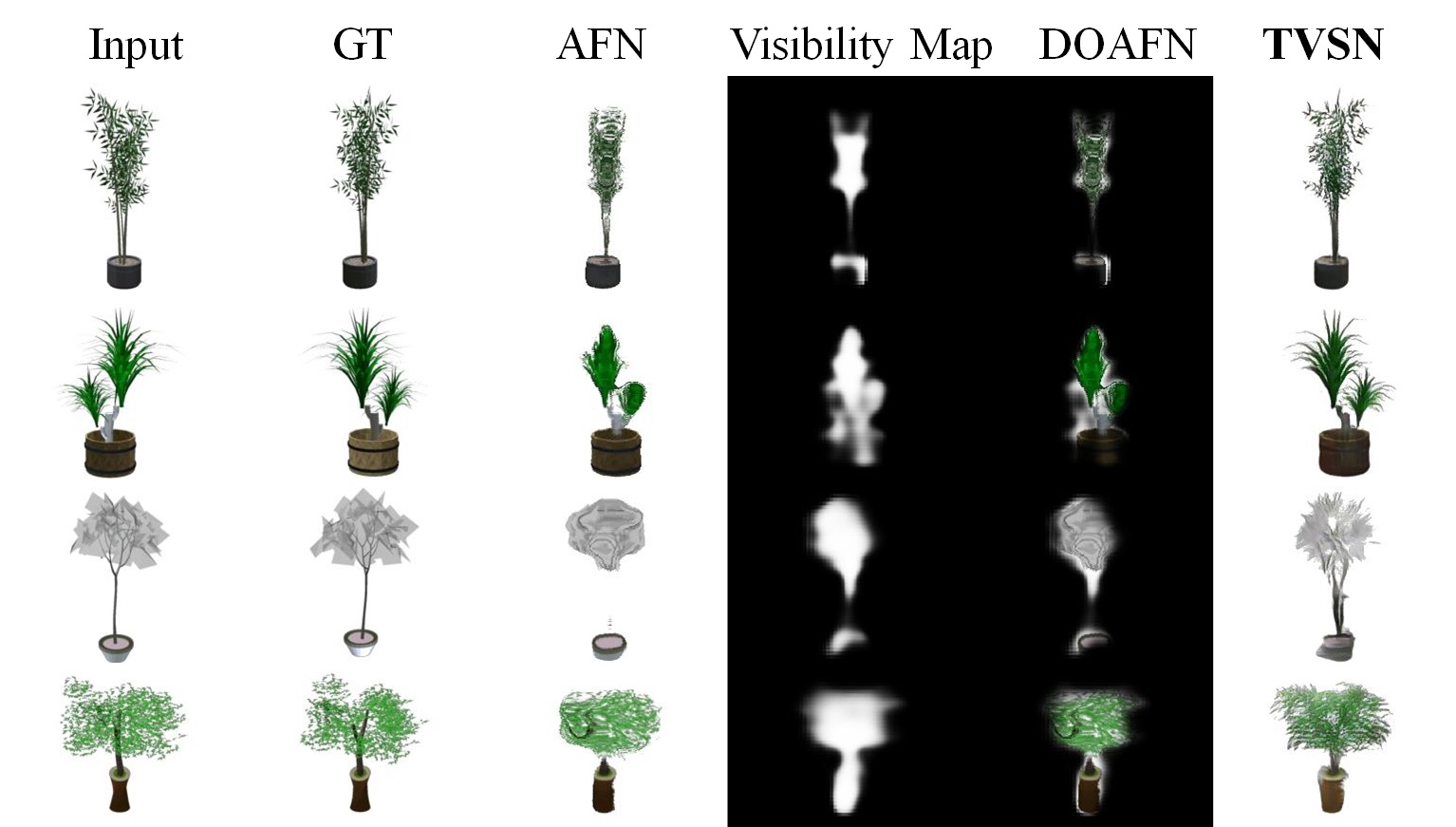
Publication
Transformation-Grounded Image Generation Network for Novel 3D View Synthesis.Eunbyung Park, Jimei Yang, Ersin Yumer, Duygu Ceylan, Alexander C. Berg
IEEE Conference on Computer Vision and Pattern Recognition (CVPR 2017)
BibTeX
@inproceedings{tvsn_cvpr2017, title = {Transformation-Grounded Image Generation Network for Novel 3D View Synthesis} author = {Eunbyung Park, Jimei Yang, Ersin Yumer, Duygu Ceylan, Alexander C. Berg}, booktitle = {The IEEE Conference on Computer Vision and Pattern Recognition (CVPR)}, month = {July}, year = {2017} }